大地の唸り Гуркіт землі
大地の唸りが真夜中に響き
Гуркіт землі лунає серед ночі,
https://note.com/poison_raika/n/n08b5d64d43c8
<>
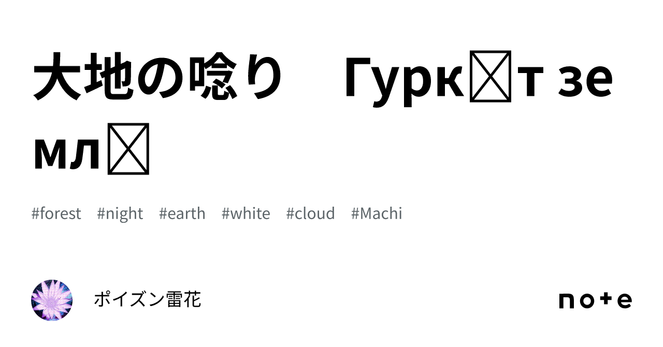
大地の唸り Гуркіт землі
大地の唸りが真夜中に響き
Гуркіт землі лунає серед ночі,
https://note.com/poison_raika/n/n08b5d64d43c8
<>
Foreign workers
Accepting immigrants is one option, but I hope it doesn't end up like Japan.
https://note.com/poison_raika/n/nf115bc62a2d0
<>
Іноземні працівники
Я сподіваюся, що прийняти іммігрантів є одним із варіантів і що ми не закінчимо, як Японія.
https://note.com/poison_raika/n/n091240368787
<>
70% Size, 100% Accuracy: Lossless LLM Compression via Dynamic-Length Float
https://arxiv.org/abs/2504.11651
#HackerNews #Lossless #LLM #Compression #Dynamic-Length #Float #AI #Research #Machine #Learning
#machine : any mechanical contrivance, as the wooden horse with which the greeks entered troy
- French: Machine
- German: die Maschine
- Italian: macchina
- Portuguese: máquina
- Spanish: máquina
------------
Fill in missing translations @ https://wordofthehour.org/r/translations
We are just biological machines who are part of ecology. There is definitely destiny. So, don't take life too seriously. Efforts, Ethics, love,skills and Moveon are ways.
Tips for Ab Crunch Machine
When done properly, the lying ab crunch isolates the rectus abdominis (your “six-pack” muscle) more effectively.
Is the #brain a #machine? Much of the enthusiasm in the so-called "AI research field" is founded on a #Nietzsche-like #atheism. But a number of researchers over the past 100 years have argued that the non-mechanical features of #quantum #coherent structures may place a role in human consciousness (which are not in the proposed 'quantum computers'). It may be a question of degree: #EEG alpha has more coherence than EEG beta, and perhaps more sensitive to tiny fluctuations eg at the quantum level.
Apple's latest "revolutionary" announcement is like a black box of buzzwords: Differential #Privacy, Aggregate Trends, and #Machine #Learning
. Apparently, they're protecting your privacy by knowing just enough about you to sell you more stuff. It's like their #innovation department found a #thesaurus and thought: "Let's make privacy sound complicated and groundbreaking!"
https://machinelearning.apple.com/research/differential-privacy-aggregate-trends #Apple #Buzzwords #HackerNews #ngated
Understanding Aggregate Trends for Apple Intelligence Using Differential Privacy
https://machinelearning.apple.com/research/differential-privacy-aggregate-trends
#HackerNews #Understanding #Aggregate #Trends #for #Apple #Intelligence #Using #Differential #Privacy
aggregate #trends #apple #intelligence #differential #privacy #machine #learning
Marriage is like a game of Jenga, you start with a solid foundation and then take turns removing pieces until it all comes crashing down, but at least the kids will have a good story to tell in therapy.
Kate Faucett &Amp; Sara Jay Take Turns 2 Fuck That Big Sex Machine!
https://s.analcity.com/3ZTI94
In case you're not from around, German lottery machine refers to a concrete mixer, because that's how they worked:
You're giving away your IP address, and therefore your location, freely. IP address protection requires special attention when it comes to improving your privacy. This is where a virtual machine can be very useful.
#privacy #ipaddress #virtual #machine
https://www.pcworld.com/article/2660359/surf-anonymously-covering-your-tracks-with-tor-tails-and-co.html
AI hasn't killed radiology, but it is changing it
https://www.washingtonpost.com/health/2025/04/05/ai-machine-learning-radiology-software/
food extruder machine for fish, cat and dog #machine #manufacturing #fishfeedmachine #petfoodmachine